HOPPER - der Online-Qualitätsoptimierer
Mit automatisch generierten Vorschlägen zu Parameter-Optimierungen reduzieren Sie Ausschuss und Zykluszeit im Spritzgießprozess.
.webp)
Reduzieren Sie Ausschuss im Spritzgießprozess - mit Hopper!
Unsere KI-Modelle lernen kontinuierlich die optimalen Prozessparameter, um den Produktionsprozess jederzeit an veränderte Rahmenbedingungen anzupassen.
Lassen Sie sich Parameter-Anpassungen automatisch vorschlagen
Hopper analysiert kontinuierlich die Einflussgrößen des Spritzgießprozesses und schlägt situativ eine Anpassung der Einstellparameter vor - mit dem Ziel, Ausschuss und Zykluszeit so gering wie möglich zu halten.
- Berücksichtigung von Material-, Prozess- und Umgebungsdaten
- Erhalten Sie Empfehlungen im Browser oder auf einem mobilen Endgerät per Push-Nachricht
= Kontinuierliche Reduzierung und Stabilisierung der Ausschussrate und Zykluszeit

Unsere KI-Modelle lernen kontinuierlich hinzu
Hopper verfolgt die Einflussgrößen des Spritzgießprozesses kontinuierlich und passt mit einem (virtuellen) Qualitäts-Label die Optimierungsmodelle kontinuierlich situativ an.
Dadurch kann auch auf veränderte Rahmenbedingungen, wie neue Produkte oder neues Material, reagiert werden. Dabei sind die Modelle übertragbar auf gleiche Maschinen.
= Kontinuierliche Reduzierung und Stabilisierung der Ausschussrate und Zykluszeit

Closed-Loop Optimierung durch automatische Parameter-Anpassung
Wenden Sie Vorschläge zur Parameteranpassung direkt an - Hopper schreibt die Parameter direkt auf die Maschinensteuerung.
Dadurch entfällt das manuelle Anpassen durch einen Maschinenbediener.
- Automatisches Anwenden von Parameteranpassungen
- Verifikation durch Nutzer erforderlich
= Reduzierung des Bedienungsaufwands von Spritzgießmaschinen

Vorteile von Hopper
5-20 %
Reduzierung von Aussschuss
Bis zu 20%
Reduktion der Zykluszeit bzw. Prozessphase
10 – 30 %
Erweiterung der Mehrmaschinenbedienung
Diese Produktionsprobleme löst Hopper.
- Optimale Einstellparameter im Elastomer- und Thermoplast-Spritzgießen sowie bei schwierig verarbeitbaren Materialen, wie z.B. bei hohen Rezyklat-Anteilen.
- Situative Reaktion auf Rohmaterial-Chargenschwankungen z.B. bei unterschiedlicher Trocknung, Temperatur, MFI-Veränderung, bei Rezyklaten (PCR) oder unterschiedlichen Elastomer-Batches.
- Vereinfachung Mehrmaschinenbedienung durch situative Vorschläge für alle angeschlossenen Maschinen

Alle Features von Hopper
Einstellparameter-Optimierung
Einstellparameter-Optimierung
Hopper analysiert kontinuierlich die Einflussgrößen des Spritzgießprozesses und schlägt situativ eine Anpassung der Einstellparameter vor - mit dem Ziel, Ausschuss und Zykluszeit so gering wie möglich zu halten.
- Berücksichtigung von Material-, Prozess- und Umgebungsdaten
- Erhalten Sie Empfehlungen im Browser oder auf einem mobilen Endgerät per Push-Nachricht

Rezept-Vergleich
Rezept-Vergleich
Vergleichen Sie angewandte Rezepte hinsichtlich KPIs und unterschiedlichen Einstellparametern.
Erhalten Sie so einen Überblick, welche Rezepte wie gut funktioniert haben.

Ausschuss-Auswertung
Ausschuss-Auswertung
Analysieren Sie auftretenden Ausschuss im Spritzgießprozess aufgeteilt nach Ausschussgründen und Kavitäten.
So können Sie Problembereiche genau identifizieren und Optimierungsmaßnahmen passgenau einleiten.
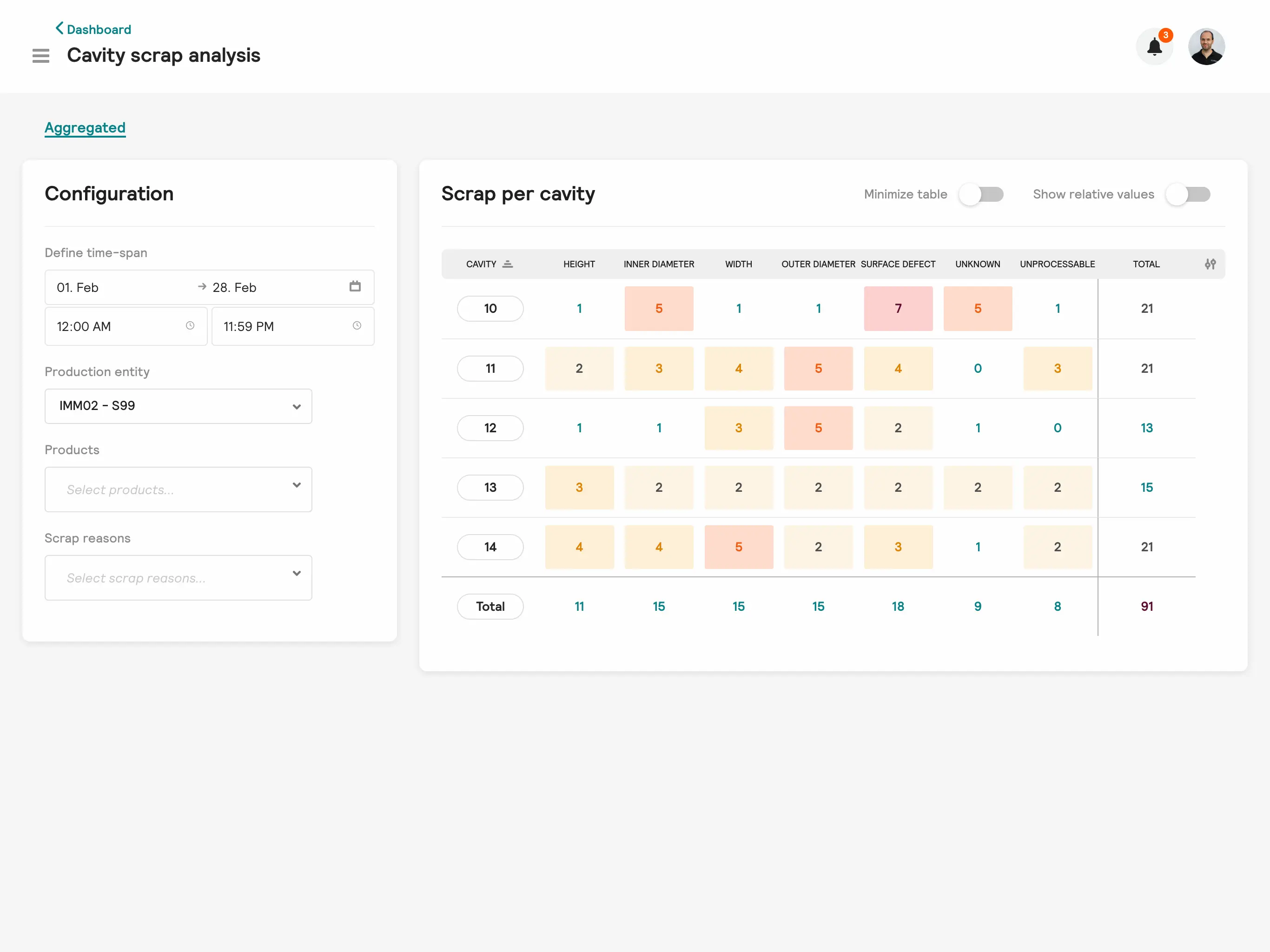
Automatische Anwendung von Parameter-Anpassungen
Automatische Anwendung von Parameter-Anpassungen
Wenden Sie Vorschläge zur Parameteranpassung direkt an - Hopper schreibt die Parameter direkt auf die Maschinensteuerung.
Dadurch entfällt das manuelle Anpassen durch einen Maschinenbediener.
- Automatisches Anwenden von Parameteranpassungen
- Verifikation durch Nutzer erforderlich

Interface
Security
Add ons
Jetzt Kostenlose Problem-Evaluation anfragen.
- Produktvorstellung mit Demo
- Roadmap zur Problemlösung
- Ganzheitliche Bewertung des Ansatzes

Weitere Produkte von plus10
Sie haben Fragen? Wir haben Antworten!
Und wenn Sie weitere Fragen haben, kontaktieren Sie uns gerne.
Die plus10 Software Tools bieten bidirektionale Schnittstellen, entweder durch gemeinsame Nutzung einer Datenbank oder durch native Protokolle zu benachbarten IT-Systemen. Wir können z.B. kontinuierlich berechnete KPIs zur Leistung und zu den Ursachen austauschen und Ihre Planungs-, Ist- und Soll-Zahlen in Echtzeit und objektiv aktualisieren. Ihre Produktionsplanung und -steuerung wird durch das Feedback von Darwin, Hopper und Shannon® deutlich präziser.
Wir unterstützen derzeit verschiedene SPS/NC-Typen. Informieren Sie sich hier über die von uns unterstützten Steuerungen oder fragen Sie uns nach der Kompatibilität zu Ihrem MES-/ERP-System.
plus10 erfasst die Daten direkt von allen integrierten Maschinensteuerungen (SPS, NC), Robotersteuerungen (RC), etc. einer Produktionsmaschine oder von allen in einer Produktionslinie integrierten Steuerungen. Dazu nutzen wir unseren plus10 DataCollector, um die Daten in hoher Frequenz von allen beteiligten SPS, NC und/oder RC zu erfassen. Bislang können wir hochfrequente Maschinendaten mit verschiedenen herstellerspezifischen SPS-Protokollen erfassen und wir fügen kontinuierlich weitere Steuerungen hinzu. Informieren Sie sich hier über die von uns unterstützten Steuerungen oder fragen Sie uns nach der Kompatibilität zu Ihrem speziellen Steuerungstyp.
Falls Sie bereits eine leistungsfähige IT-Infrastruktur in Ihrer Fabrik installiert haben, bieten wir auch die Anbindung an Ihre Middleware an.
Grundsätzlich erfasst und verarbeitet plus10 alle Daten der Maschinensteuerung (SPS) der Produktionsmaschinen oder der Produktionslinie in hoher Frequenz. Die hohe Abtastrate (wir sprechen von Millisekunden) ist notwendig, um die Ursachen von Problemen und nicht nur deren Folgeerscheinungen zu erkennen und zuzuordnen. In einigen Fällen werden zusätzlich die RC- und NC-Daten von z.B. Handlingrobotern benötigt. Im Standardfall müssen keine zusätzlichen Sensoren oder Kameras installiert werden.
Die plus10 Software-Tools sind flexibel in Bezug auf die IT-Infrastruktur. Die Datenerfassung und Vorverarbeitung läuft kontinuierlich lokal, z.B. auf einem Industrial Edge Device oder einer virtuellen Maschine innerhalb des Maschinensteuerungsnetzwerks. Für den nächsten Verarbeitungsschritt passt sich plus10 an Ihre bevorzugte Lösung an: Wir können eine Cloud-Umgebung für unsere Software-Tools anbieten oder sie werden auf einer virtuellen Maschine on-premise in Ihrem bestehenden werksinternen Rechenzentrum ausgeführt. Beides ist möglich und wir haben Erfahrung mit beiden Optionen.
Shannon®, Hopper und Darwin sind als Software-as-a-Service verfügbar und werden auf Microsoft Azure im plus10-Abonnement gehostet. Darüber hinaus kann unsere Software in einer kundenspezifischen Cloud-Infrastruktur oder als On-Premise-Lösung betrieben werden.
Für Shannon® gibt es Apps für Android und iOS (inkl. watchOS).
Unsere jährliche Lizenzgebühr beinhaltet Standard-Support und Software-Updates auf die neueste Version des/der bestellten Software-Service(s). Mit einer plus10 Softwarelizenz von Darwin, Hopper oder Shannon® garantieren wir Ihnen also die neueste, effizienteste und benutzerfreundlichste Version unserer Optimierungssoftware. Dabei betreuen wir weltweit Produktionsanlagen, in denen unsere Software Tools installiert sind.
Dies hängt von der Art der Maschinensteuerung (SPS) und der vorhandenen IT-Infrastruktur ab. Wenn die Konnektivität zu allen SPS besteht und Sie die Recheninfrastruktur bereitstellen (entweder als lokale virtuelle Maschine oder als dezidierter Server), kann die Installation und Konfiguration remote durchgeführt werden. In diesem Fall wird die Schulung des Bedienpersonals auf Shannon®, Hopper oder Darwin immer noch vor Ort durchgeführt, kann aber als Video-Schulung virtualisiert werden, auch im Falle von pandemischen Schließungen oder Zugangsbeschränkungen.
Alle plus10-Software Tools dienen der Bewältigung eines hohen Komplexitätsgrads in der Produktion im Mehrschichtbetrieb.
Je nach Bedarf können Sie ein geeignetes plus10-Tool auswählen, um Ihre spezifischen Produktionsprobleme zu bewältigen:
- Shannon® hilft Ihnen bei Störungen und technischen Ausfällen. und optimiert so die technische Verfügbarkeit.
- Darwin erkennt kontinuierlich Leistungsgrad-Optimierungspotenziale während des Betriebs – entweder in verketteten und automatisierten Prozessketten oder bei mindestens 6 gleichen Einzelmaschinen. Diese Maschinen oder Produktionslinien können auch in verschiedenen Werken auf der ganzen Welt stehen.
- Hopper senkt Ihre Ausschussrate in Kombination mit der Zykluszeit. Das heißt Hopper hilft, hohe Ausschussraten oder Konformitätskosten zu reduzieren und optimiert so die Qualität.
Alle plus10-Softwaretools können nur auf vollautomatisierten Produktionsmaschinen oder Produktions-/Montagelinien eingesetzt werden, da ein automatischer Dateninput aus jedem Prozessschritt erforderlich ist. Die Bediener/-innen übernehmen in der Regel logistische und unterstützende Aufgaben wie das Befüllen oder die Entnahme von Material sowie statistische Prozesskontrollen und die Fehlersuche im Falle von Problemen.